Agentic AI vs Traditional AI: Key Differences Explained
AI
Understanding Agentic AI and Traditional AI
Artificial Intelligence (AI) has become an integral part of modern technology, influencing everything from how we work to how we interact with devices. However, not all AI is created equal. Two prominent categories of AI are Agentic AI and Traditional AI, each with distinct characteristics and applications.
Agentic AI refers to systems designed to perform tasks with a certain level of autonomy, decision-making capabilities, and adaptability. These systems can interact with their environment and make informed decisions without constant human intervention. On the other hand, Traditional AI typically comprises systems that follow predefined rules and algorithms, lacking the ability to adapt to new situations independently.

The Core Difference: Autonomy
One of the most significant differences between Agentic AI and Traditional AI is their level of autonomy. Agentic AI is built to perform tasks autonomously by leveraging advanced machine learning techniques and neural networks. These systems can learn from their experiences, improving their performance over time without additional programming.
In contrast, Traditional AI systems are more rigid. They rely heavily on human input for updates and improvements, as they follow a fixed set of instructions or algorithms. While they can process data and execute tasks efficiently, their lack of adaptability makes them less suitable for dynamic environments.
Applications and Use Cases
Agentic AI has found its place in various industries where adaptability and decision-making are crucial. For example, autonomous vehicles use Agentic AI to navigate roads, recognize obstacles, and make real-time driving decisions. In finance, these systems can predict market trends and optimize trading strategies based on current data.
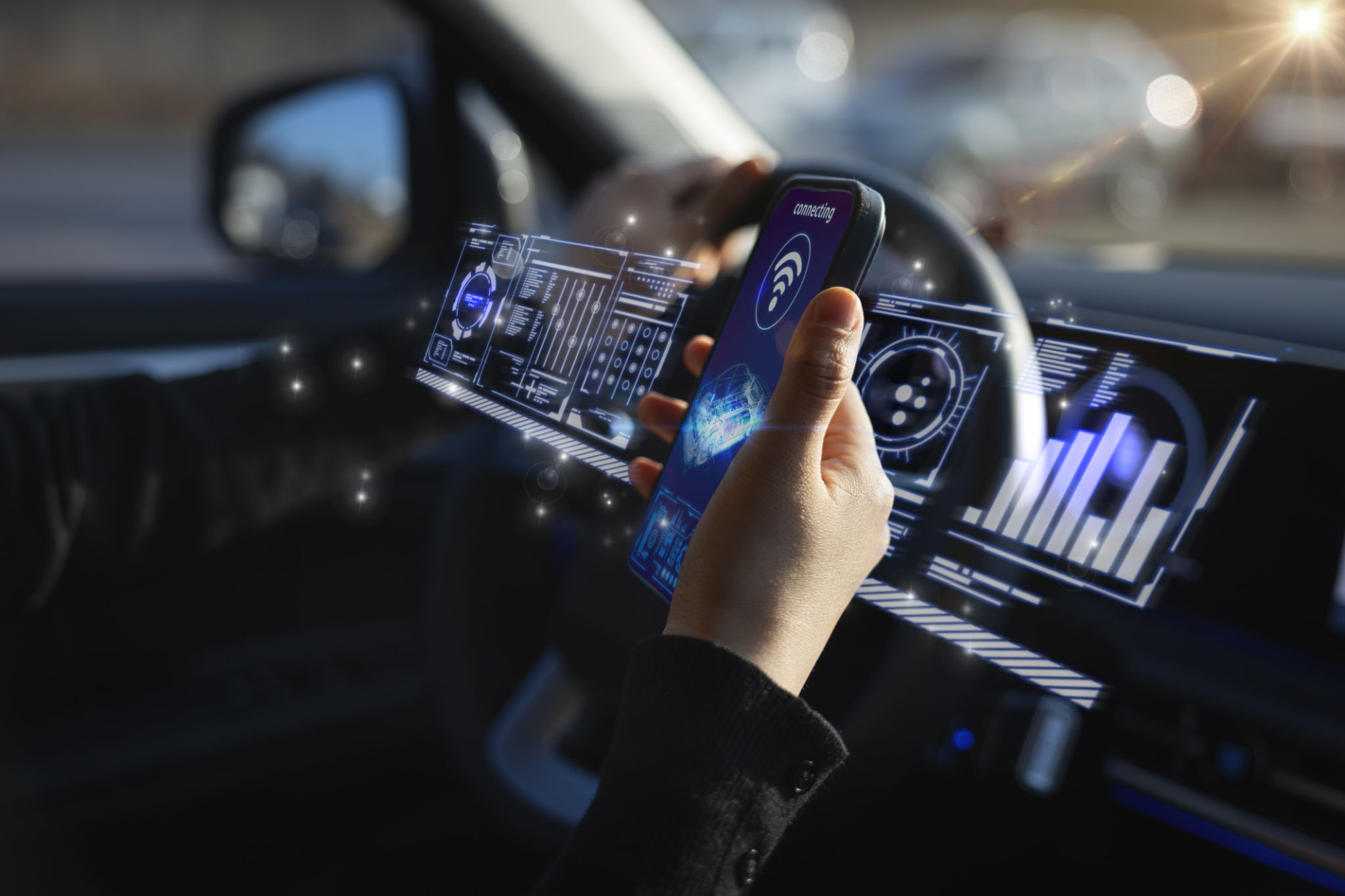
Traditional AI, however, is often used in scenarios where tasks are repetitive and require consistency. Examples include data entry automation, chatbots for customer service with predefined responses, and basic data analysis tasks. These applications benefit from the reliability and predictability of Traditional AI systems.
Challenges and Limitations
Despite the advancements in Agentic AI, there are challenges associated with its implementation. Ensuring the ethical use of autonomous systems and maintaining control over their actions are significant concerns. Moreover, the complexity involved in developing these systems demands substantial resources and expertise.
Traditional AI systems, while easier to implement, are limited by their lack of flexibility. They can struggle to perform effectively in environments that require adaptation or complex decision-making. This limitation can hinder their applicability in rapidly changing industries.
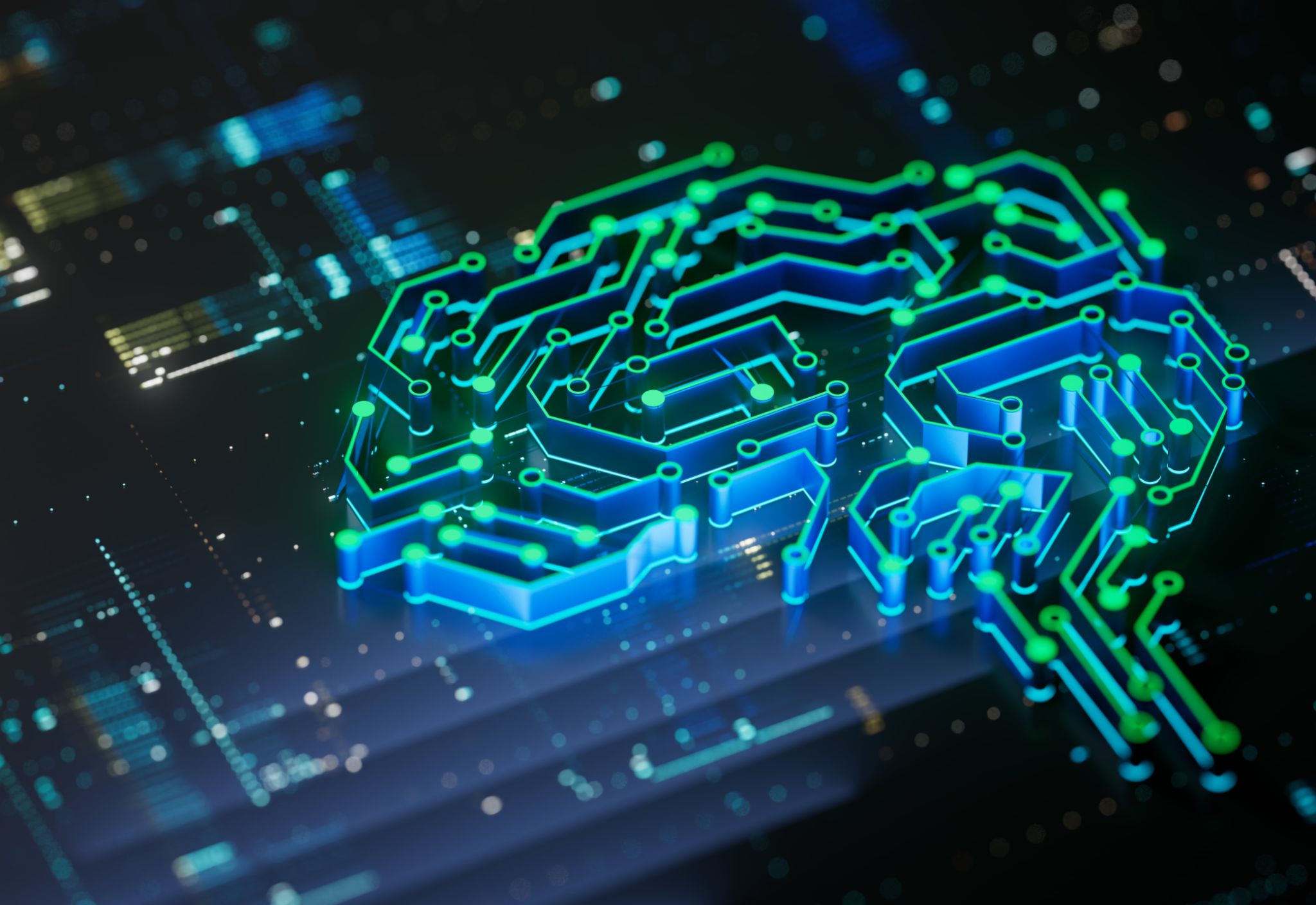
The Future of AI
The future of AI likely involves a blend of both Agentic and Traditional approaches. The integration of these technologies can lead to systems that benefit from both adaptability and reliability. As research continues, it's anticipated that Agentic AI will become more accessible, allowing its deployment across a broader range of sectors.
In summary, understanding the differences between Agentic AI and Traditional AI is crucial for leveraging their strengths effectively. Whether it's enhancing autonomy or ensuring reliability, each type of AI has its own role in shaping the technological landscape of tomorrow.